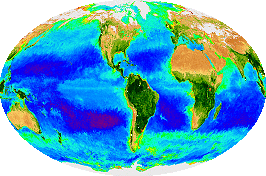
Satellite ocean color has a spectroscopic capability to estimate Chlorophyll-a (Chl-a) concentration on the ocean surface. Chl-a is estimated as a proxy to determine the phytoplankton biomass distribution and becomes an indicator of the trophic status of the water body especially Harmful Algae Bloom (HAB).

Long-term records of ocean color data at lower spatial resolution has been used widely and offered the opportunity for the development of ocean colour algorithms. Most of the ocean color algorithms performed well in a clear water state, but derived significant uncertainty in algae prone area especially at near coast and shallow water using coarse spatial resolution image.

This study demonstrate the innovative method of ocean colour retrieval by machine learning modeling. Chlorophyll-a and nutrient measurement from sea-truth were used.

Results suggested that powerful capability of the machine learning approach has improve the ocean color algorithm accuracy which is limited by the complex optical interaction in coastal waters.