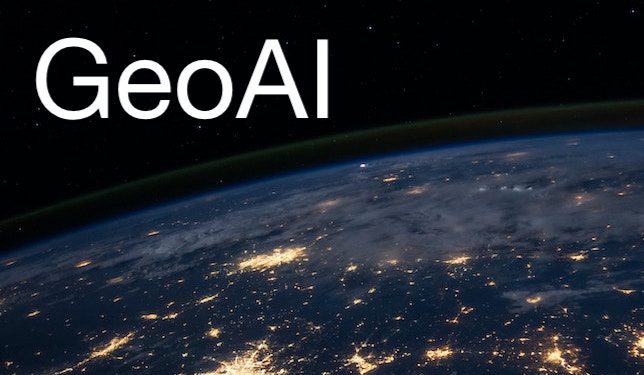
By Shahabuddin Amerudin
Introduction
The term Geospatial Artificial Intelligence (GeoAI) lacks a universally agreed-upon definition. Initially, GeoAI referred to the utilisation of machine learning tools within Geographic Information Systems (GISs) to predict future scenarios by classifying data. This included disaster occurrence, human health epidemiology, and ecosystem evolution, aimed at bolstering community resilience through traditional geographic information in digital cartography (Esri, 2018). A broader interpretation considers GeoAI as processing Geospatial Big Data (GBD) encompassing various sources, such as digital cartography, remote-sensing-based multidimensional data, and georeferenced texts. The focal point is the geographic dimension (Janowicz et al., 2019. Thus, GeoAI merges AI techniques and data science with GBD to comprehend natural and social phenomena. A comprehensive definition views GeoAI as utilizing artificial intelligence methods like machine learning and deep learning to extract insights from spatial data and imagery (Hernandez, 2020). GeoAI serves as an emerging analytical framework, facilitating data-intensive geographic information science and environmental and social sensing, thereby understanding human mobility patterns and societal dynamics.
GeoAI’s Challenges and Research Topics
The distinctive geospatial dimension, conceptual diversity between “place” and “space,” varied spatial information formats, and diverse scales create challenges and opportunities for GeoAI (Bordogna and Fugazza, 2023). Addressing the unique geosemantics and analytical needs dictated by application goals poses new hurdles with AI integration. Research directions encompass topics like multi-resolution GBD fusion, multi-source data integration, geosummarization for enhanced data quality, and deep learning exploration in remote sensing imagery (CNN, RCNN, LSTM, GANs) (Janowicz et al., 2019). A crucial goal is bridging the gap between complex AI technologies like deep learning and transparent methods such as decision trees, clustering, and data mining. This convergence can promote explainable AI features, critical for safety-critical domains like healthcare and law enforcement.
GeoAI for Analyzing Geotagged User-Generated Content and Traces
This section delves into innovative approaches to classify and mine geotagged user-generated content and traces within social networks:
- In the study “Spatio-Temporal Sentiment Mining of COVID-19 Arabic Social Media” by Elsaka et al. (2022), diverse AI techniques combine NLP and GeoAI to analyze geotagged Arabic tweets addressing the COVID-19 pandemic. Techniques for inferring geospatial data from non-geotagged tweets were developed, followed by sentiment analysis at various location resolutions and topic abstraction levels. Correlation-based analysis between Arabic tweets and official health data was also presented. Results indicated enhanced location-enabled tweets (from 2% to 46%) and identified correlations between topics like lockdowns, vaccines, and COVID-19 cases. The study underscores social media’s role as a valuable “social sensing” tool.
- “Automatic Classification of Photos by Tourist Attractions Using Deep Learning Model and Image Feature Vector Clustering” by Kim and Kang (2022) exemplifies social sensing. The study automates the classification of tourist photos based on attractions using deep learning and image feature clustering. The method, applied to TripAdvisor photos, offers flexibility in extracting categories for each destination and robust classification performance with limited data.
- “Detecting People on the Street and the Streetscape Physical Environment from Baidu Street View Images and Their Effects on Community-Level Street Crime in a Chinese City” by Yue et al. (2022) showcases social sensing’s potential. Utilizing Baidu Street View images, deep learning, and spatial statistical regression models, the study assesses street crime through user traces. This pioneering approach quantifies street inhabitants and streetscape features impacting crime, revealing the positive correlation between street population and crime assessments.
Discussion
The evolution of GeoAI has illuminated its pivotal role in unraveling complex spatial phenomena and providing valuable insights across diverse domains. The multifaceted definitions of GeoAI reflect its adaptability to a wide range of applications, from predicting disasters and tracking health trends to understanding human mobility patterns through social sensing. This adaptability, however, presents challenges related to the uniqueness of geospatial data, the heterogeneity of spatial information, and the need for transparent AI solutions.
One of the key takeaways from the exploration of GeoAI’s applications is its capacity to extract actionable insights from geotagged user-generated content and traces. The studies discussed shed light on the potency of combining advanced AI techniques with geospatial data to tackle real-world challenges. For instance, the analysis of Arabic tweets during the COVID-19 pandemic not only improved geotagging accuracy but also revealed correlations between sentiment and health outcomes. Similarly, the automatic classification of tourist photos based on attractions exemplified how GeoAI can contribute to enhancing the tourism experience through personalized recommendations.
Furthermore, the discussion around the use of GeoAI in assessing street crime via user traces demonstrates the potential of AI to leverage previously untapped data sources. By harnessing Baidu Street View images and deep learning, researchers were able to quantify the relationship between street population and crime assessments. This underscores the transformative potential of GeoAI in contributing to urban planning, crime prevention, and public safety.
Conclusion
In conclusion, Geospatial Artificial Intelligence (GeoAI) presents an exciting frontier for innovation and understanding across various domains. Its ability to analyze spatial data, extract patterns from geotagged content, and predict future scenarios is reshaping how we approach complex challenges. GeoAI’s versatility, as showcased through applications like sentiment analysis during the pandemic, tourist attraction classification, and crime assessment, underscores its potential to drive positive change in society.
However, the journey of GeoAI is not without obstacles. The diversity of geospatial data sources, the need for transparent and explainable AI models, and the integration of multi-source data pose challenges that require ongoing research and development. As GeoAI continues to advance, striking a balance between harnessing the power of complex AI techniques and ensuring interpretability and accountability becomes crucial.
Ultimately, GeoAI’s evolution will rely on collaborative efforts between AI experts, geospatial specialists, domain experts, and policymakers. By combining their expertise, we can navigate the intricate landscape of GeoAI, harnessing its potential to create a safer, more sustainable, and more informed world. Through continued exploration, research, and refinement, GeoAI is poised to revolutionize how we understand and interact with the intricate spatial dynamics of our planet.
References
Bordogna, G. and Fugazza, C. (2023). Artificial Intelligence for Multisource Geospatial Information. ISPRS Int. J. Geo-Inf., 12, 10. https://doi.org/10.3390/ijgi12010010
Elsaka, T., Afyouni, I., Hashem, I., and Al Aghbari, Z. (2022). Spatio-Temporal Sentiment Mining of COVID-19 Arabic Social Media. ISPRS Int. J. Geo-Inf., 11, 476.
Esri (2018). What is GeoAI? Available online: https://ecce.esri.ca/mac-blog/2018/04/23/what-is-geoai/ (accessed on 10 May 2023).
Hernandez, L. (2020). ELISEWebianar: GeoAI—Presentation: Geospatial Data and Artificial Intelligence—A Deep Dive into GeoAI. Available online: https://joinup.ec.europa.eu/collection/elise-european-location-interoperability-solutions-e-government/document/presentation-geospatial-data-and-artificial-intelligence-deep-dive-geoai (accessed on 10 May 2023).
Kim, J. and Kang, Y. (2022). Automatic Classification of Photos by Tourist Attractions Using Deep Learning Model and Image Feature Vector Clustering. ISPRS Int. J. Geo-Inf. 2022, 11, 245.
Janowicz, K., Gao, S., McKenzie, G., Hu, Y., and Bhaduri, B. (2019). GeoAI: Spatially explicit artificial intelligence techniques for geographic knowledge discovery and beyond. Int. J. Geogr. Inf. Sci., 34, 625–636.
Yue, H., Xie, H., Liu, L., and Chen, J. (2022). Detecting People on the Street and the Streetscape Physical Environment from Baidu Street View Images and Their Effects on Community-Level Street Crime in a Chinese City. ISPRS Int. J. Geo-Inf., 11, 151.
Suggestion for Citation: Amerudin, S. (2023). Unveiling the Power of Geospatial Artificial Intelligence (GeoAI) and its Applications. [Online] Available at: https://people.utm.my/shahabuddin/?p=6716 (Accessed: 21 August 2023).