By Shahabuddin Amerudin
The increasing frequency and severity of pipeline-related disasters, exemplified by the catastrophic 2025 Putra Heights gas explosion, highlight critical shortcomings in conventional infrastructure monitoring approaches. This theoretical framework presents a comprehensive paradigm shift from reactive response systems to proactive, predictive geospatial intelligence systems for pipeline safety management. At its core, this framework integrates three fundamental technological domains: artificial intelligence (AI) for pattern recognition and predictive analytics, geographic information systems (GIS) for spatial contextualization and visualization, and Internet of Things (IoT) sensor networks for real-time data acquisition.
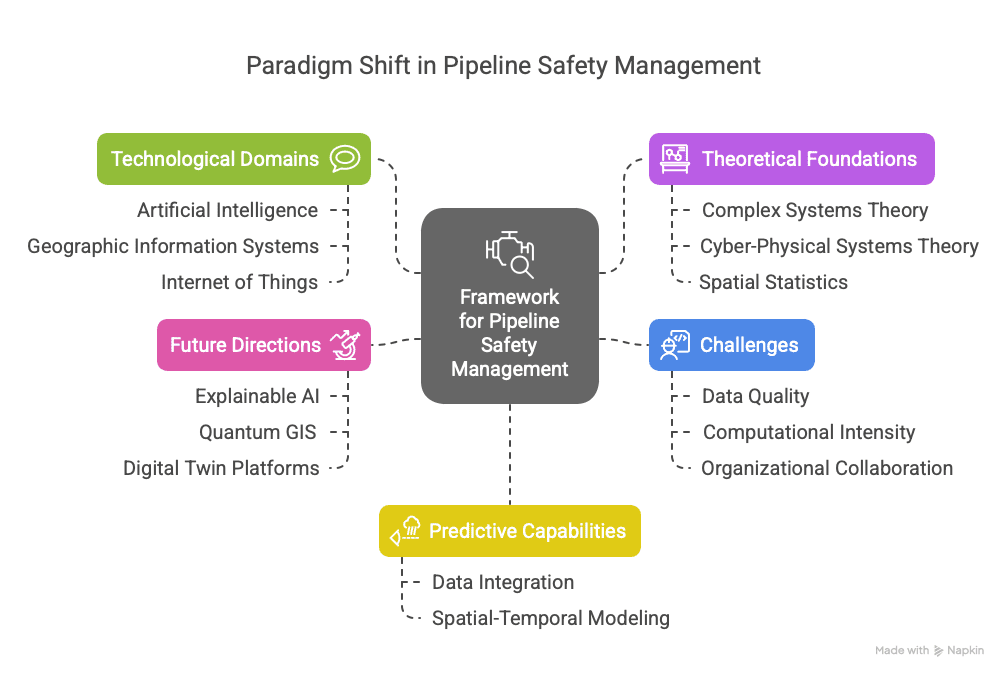
The theoretical foundation of this framework draws upon several interconnected disciplines. From complex systems theory, we understand that pipeline networks represent nonlinear dynamical systems where small perturbations can lead to catastrophic failures through cascading effects. Cyber-physical systems theory provides the conceptual basis for digital twin technology, enabling the creation of virtual replicas that mirror physical pipeline infrastructure in real-time. Spatial statistics and geocomputation principles underpin the predictive modeling capabilities, allowing for the identification of high-risk zones based on spatial autocorrelation patterns and terrain analysis.
The framework’s predictive capabilities are enabled through multi-layered data integration. At the base level, distributed IoT sensors continuously monitor pipeline integrity parameters including pressure fluctuations, temperature variations, vibration patterns, and corrosion rates. Middleware layers process this streaming data using edge computing architectures to reduce latency, while cloud-based platforms perform large-scale spatial analytics. Machine learning algorithms, particularly deep neural networks and ensemble methods, analyze both real-time sensor data and historical incident records to identify precursor patterns associated with impending failures.
A critical innovation of this framework is its spatial-temporal predictive modeling approach. By incorporating time-series analysis of geospatial data, the system can forecast not just where failures are likely to occur, but when they might happen. This temporal dimension is particularly valuable for scheduling preventive maintenance and optimizing resource allocation. The models integrate diverse data sources including soil composition maps, seismic activity records, urban development patterns, and climate projections to create comprehensive risk assessments.
Implementation of this framework faces several technical and organizational challenges that require careful consideration. Data quality and sensor reliability issues must be addressed through robust calibration protocols and redundant monitoring systems. The computational intensity of real-time predictive analytics necessitates distributed computing architectures and potentially quantum computing solutions for large-scale pipeline networks. Perhaps most significantly, successful deployment requires breaking down institutional silos between utility companies, municipal planners, and emergency response agencies to enable seamless data sharing and coordinated action.
The framework’s validation and continuous improvement are achieved through a closed-loop learning system. Each predicted event and actual incident provides new data that refines the machine learning models, creating a positive feedback cycle that enhances predictive accuracy over time. This adaptive capability is particularly important in the context of climate change, where evolving weather patterns and extreme events may alter failure mechanisms in ways that historical data alone cannot anticipate.
Looking ahead, this theoretical framework points to several promising research directions. The integration of explainable AI techniques will be crucial for building trust in predictive systems among stakeholders and regulators. Advances in quantum GIS could dramatically accelerate the processing of large geospatial datasets, enabling near-instantaneous risk assessments. Furthermore, the development of standardized digital twin platforms will facilitate interoperability between different pipeline networks and municipal infrastructure systems.
Ultimately, this framework represents more than just a technological upgrade – it embodies a fundamental transformation in how we conceptualize and manage critical infrastructure. By shifting from a paradigm of periodic inspections and post-failure analysis to one of continuous monitoring and predictive prevention, we can significantly enhance public safety while optimizing maintenance resources. The implementation of such systems will require substantial investment and organisational change, but the potential benefits in terms of lives saved, environmental protection, and economic resilience make this transformation both necessary and urgent for modern infrastructure management.